From Superinnovators 22/08/23
In a groundbreaking experiment, Google’s research team has successfully deployed a fleet of 23 reinforcement learning (RL) enabled robots to sort waste and recycling in office buildings.
The experiment, which spanned over two years, was led by Sergey Levine, Research Scientist, and Alexander Herzog, Staff Research Software Engineer at Google Research’s Brain Team.
Reinforcement learning has been a promising field in robotics.
It enables robots to learn complex behaviours through trial-and-error interactions.
While RL has been used to teach robots skills like grasping and playing table tennis, its application in real-world settings has been limited.
This is due to the complexity and diversity of the real world.
The project titled “Deep RL at Scale: Sorting Waste in Office Buildings with a Fleet of Mobile Manipulators” aimed to tackle this challenge.
The robots were deployed in Google office buildings to sort waste and recycling.
They used scalable deep RL combined with simulation training and auxiliary object perception inputs.
The system was validated with 4,800 evaluation trials across 240 waste station configurations.
The problem of improper waste sorting is significant.
It leads to contamination of recyclables and improper disposal of compost.
Google’s robots were tasked with sorting waste into appropriate bins for recyclables, compost, and landfill trash.
The task was complex due to the variety of objects and the need to sort them quickly and efficiently.
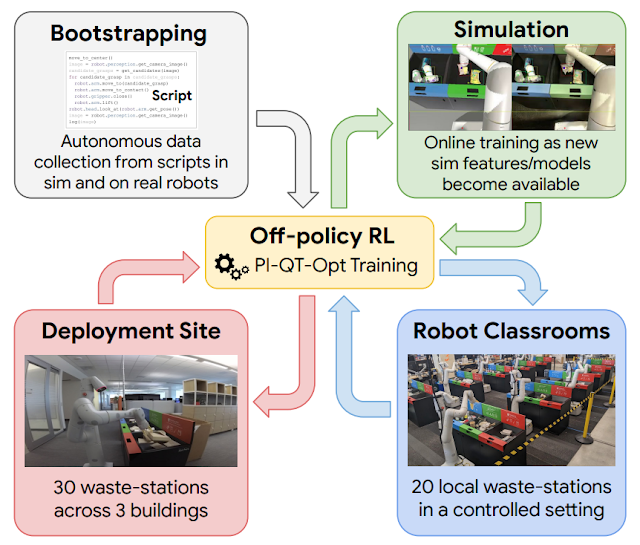
To enable the robots to learn, four sources of experience were used.
These included simple hand-designed policies, simulated training, practice in “robot classrooms,” and real deployment in office buildings.
The RL framework was based on QT-Opt, previously applied in laboratory settings.
It used a CycleGAN-based transfer method to make simulated images appear more life-like.
The robots trained in classrooms and real office buildings.
They gathered 540k trials in classrooms and 32.5k trials from deployment.
The final system could accurately sort about 84% of the objects.
This reduced contamination in waste bins by 40% to 50% by weight.
The success of this experiment demonstrates the potential of RL-based systems in real-world tasks.
The combination of offline and online data enabled the robots to adapt to various real-world situations.
Yet, there is still room for improvement.
The team is looking at larger models and other sources of experience to enhance performance.
Google’s experiment with RL-enabled robots for waste sorting marks a significant step forward in the application of robotics in everyday settings.
The blend of simulation, classroom learning, and real-world deployment offers a promising pathway for future developments in robotics.