From Hefei Institutes of Physical Science, Chinese Academy of Sciences 25/08/23
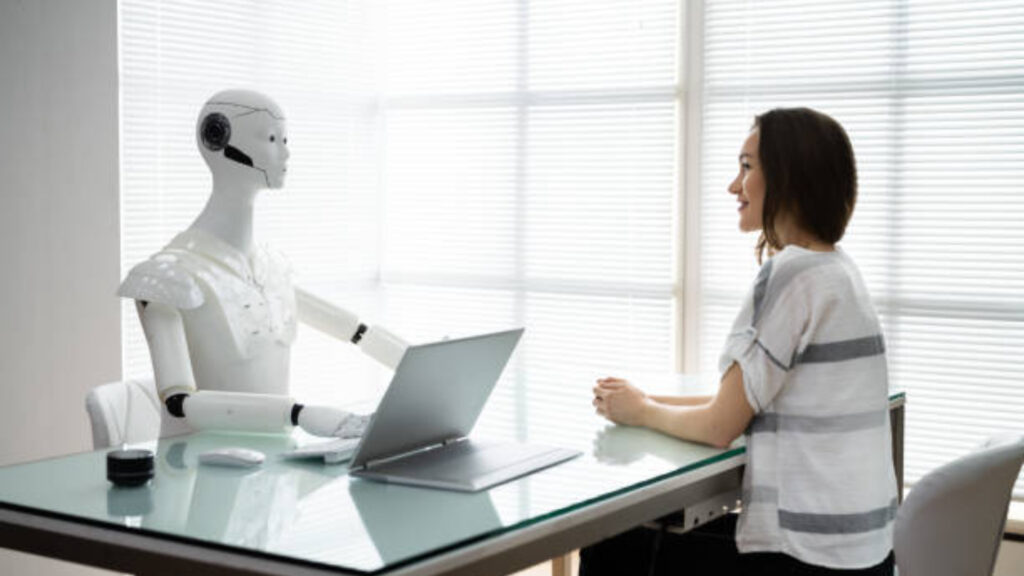
A research team led by Prof. LI Hai from Hefei Institutes of Physical Science (HFIPS), Chinese Academy of Sciences (CAS) concluded their research in AI-powered language assessment for cognitive and motor disorders field in a series of paper recently.
Acoustic and linguistic features in speech are sensitive markers of brain disorders and aging enabling automated cognitive evaluation speech assessment possible.
In this research, the team developed an AI-powered language assessment system that utilized acoustic and linguistic features in speech to evaluate cognitive abilities automatically.
This system incorporated a standardised computerized speech task battery commonly used in clinical settings, a machine learning module, and an automated scoring and reporting system.
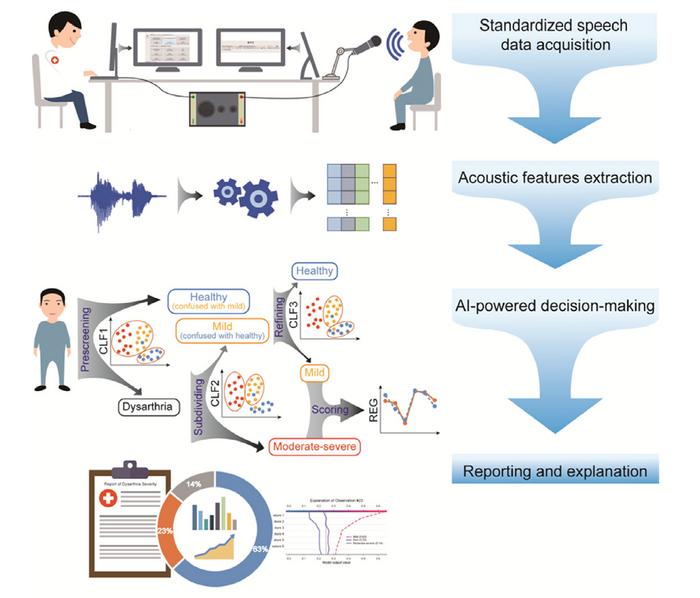
In their paper published in Computer Speech & Language, the team conducted a comprehensive investigation into the reliability of the speech task battery across seven cognitive tasks and around a hundred speech acoustic features.
This research provided crucial data support for feature engineering.
To address the methodological challenge of assessing the reliability of online tests, the team also refined a split-half reliability estimation method that was published in Behavior Research Method.
The clinical effectiveness of the AI-powered language assessment system was validated using a valuable sample of individuals with Wilson Disease (WD), which served as an ideal disease model with mixed dysarthria phenotypes.
The team demonstrated the superior performance of their model in predicting dysarthria severity in a recent paper published in Advanced Intelligent Systems.
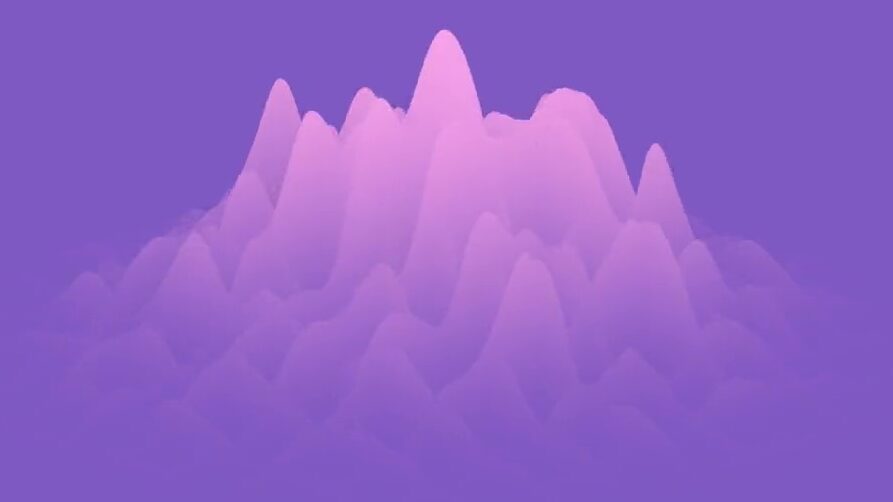
Their systematic methodological framework provided valuable insights into AI-driven intelligent diagnosis, rehabilitation, and home support for neurological diseases.
Furthermore, the team explored the feasibility of automated assessment for cognitive disorders, including dementia.
They examined the role of automatic speech recognition (ASR) in dementia detection and verified the effectiveness of an end-to-end prediction model for dementia detection based on various commercial ASR tools.
The results of this study were published in Dementia and Geriatric Cognitive Disorders.
This series of research advancements highlighted the successful interdisciplinary collaboration among computer scientists, neurology clinicians, and cognitive psychologists, establishing a promising translational medicine model.